Emilia Matos do Nascimento1,2, Clarisse Lopes de Castro Lobo2, Basilio de Bragança Pereira3,4,5 and Samir K. Ballas2,6.
1 UEZO - Centro Universitário Estadual da Zona Oeste, Rio de Janeiro, RJ, Brazil.
2 Clinical Hematology Division, Instituto de Hematologia Arthur de Siqueira Cavalcanti. HEMORIO, Rio de Janeiro, RJ, Brazil.
3 School of Medicine/UFRJ - Federal University of Rio de Janeiro, RJ, Brazil.
4 Postgraduate School of Engineering – COPPE/UFRJ.
5 Clementino Fraga Filho University Hospital/UFRJ.
6 Cardeza Foundation, Department of Medicine, Jefferson Medical College, Thomas Jefferson University, Philadelphia, PA, USA.
Correspondence to: Samir K. Ballas MD FACP. Cardeza Foundation,
Department of Medicine, Jefferson Medical College, Thomas Jefferson
University, 1020 Locust Street, Philadelphia, PA 19107. Tel: 856 745
6380, Fax: 856 795 0809. E-Mail:
Samir.ballas@jefferson.edu
Published: March 1, 2019
Received: November 12, 2018
Accepted: January 12, 2019
Mediterr J Hematol Infect Dis 2019, 11(1): e2019022 DOI
10.4084/MJHID.2019.022
This is an Open Access article distributed
under the terms of the Creative Commons Attribution License
(https://creativecommons.org/licenses/by-nc/4.0),
which permits unrestricted use, distribution, and reproduction in any
medium, provided the original work is properly cited.
|
Abstract
The
clinical picture of patients with sickle cell anemia (SCA) is
associated with several complications some of which could be fatal. The
objective of this study is to analyze the causes of death and the
effect of sex and age on survival of Brazilian patients with SCA. Data
of patients with SCA who were seen and followed at HEMORIO for 15 years
were retrospectively collected and analyzed. Statistical modeling was
performed using survival analysis in the presence of competing risks
estimating the covariate effects on a sub-distribution hazard function.
Eight models were implemented, one for each cause of death. The
cause‐specific cumulative incidence function was also estimated. Males
were most vulnerable for death from chronic organ damage (p = 0.0005)
while females were most vulnerable for infection (p=0.03). Age was
significantly associated (p ≤ 0.05) with death due to acute chest
syndrome (ACS), infection, and death during crisis. The lower survival
was related to death from infection, followed by death due to ACS. The
independent variables age and sex were significantly associated with
ACS, infection, chronic organ damage and death during crisis. These
data could help Brazilian authorities strengthen public policies to
protect this vulnerable population.
|
Introduction
The
clinical picture of patients with sickle cell anemia (SCA) is
associated with several complications some of which could be fatal.[1-3]
Besides the recurrent episodes of acute vaso-occlusive crises (VOCs),
these complications include, among other things, stroke, acute chest
syndrome, splenic and hepatic sequestration, infections, priapism, leg
ulcers, retinopathy, avascular necrosis, cholelithiasis, progressive
organ failure and death.[3] The frequency and severity
of these complications vary with age, time and sex. Ischemic stroke,
for example, is more common in children whereas leg ulcers are more
common in adults.[3] The probability of the recurrence
of each complication at a certain age has not been well studied. The
objective of this study is to analyze the causes of death using the
competitive risk statistical model.[4] This analysis
is based on the recently reported retrospective data on the pattern of
morbidity and mortality in children, adolescents and adults in Rio de
Janeiro, Brazil.[5]
Material and Methods
Patients: Retrospective data were collected and analyzed in patients with SCA including patients with sickle-β0-thalassemia (Sβ0thal),
who were seen and followed at HEMORIO for 15 years from January 1,
1998, through December 31, 2012. Children and adults were included in
the study. The total number of patients enrolled was 1676. The
diagnosis of SCA including sickle-β0-thalassemia
was confirmed by high-performance liquid chromatography (HPLC). The
date and cause of death were confirmed from the patients’ charts if
death occurred at HEMORIO. Death outside HEMORIO was suspected if
patients failed to show up for follow-up and confirmed by interviews
with the patients’ families and from death certificates. None of the
patients was taking hydroxyurea. The study was approved by the
Institutional Review Board (IRB) of HEMORIO.
Statistical analysis. Statistical modeling was performed using Survival analysis in the presence of competing risks.
Basically,
three functions are used in survival data: the survival function, the
cumulative distribution function, and the hazard function.
In
survival analysis, it is common to investigate the lifetime related to
a single cause of death. However, there are more complex models, in
which the death of the individual is related to one of several possible
causes identified in the study. These models are called competing risk
models being suitable in studies where individuals are exposed to more
than one cause of failure or event.
Gooley et al.[6]
define the competing risk as a survival model in which the occurrence
of an event prevents or alters the probability of occurrence of another
event.
In general, three types of approach are used in the presence of competing risks:[6] 1 - Event-free survival model, using the Cox model[4]
considering the time until the occurrence of the first event. This
model is not suitable because it does not consider the various risk
factors; 2 – Cause-specific hazard model, where the Cox model is used
considering one of the events as the main cause and the rest are
censored. This approach is also unsuitable because it is not possible
to estimate the common effect of a covariate for competing outcomes.
Additionally, the sum of the cumulative distribution function for each
outcome is different from the cumulative distribution function of the
overall curve. It would also be necessary to be valid the assumption of
independence between the event of interest and other competing events,
considered censorship, which rarely occurs; and 3 – Hazard of
subdistribution model, using the cumulative incidence function. This
model does not require any assumption of independence of competing
risks.[7]
The cumulative incidence function, or subdistribution function, introduced by Kalbleisch & Prentice,[8] is defined as the joint probability
 |
|
That is, Fi (t) is the probability of failure for a specific cause, among p possible causes over time.
Fine and Gray[9]
proposed a regression model implemented on the cumulative incidence
function for analyzing competing risks. Modeling is performed by hazard
of subdistribution function, defined as the instantaneous hazard of an
individual suffering the event for a specific cause, conditional to
have survived until a certain time t.
Where γ is the hazard of subdistribution; γ0 is the baseline hazard of the subdistribution; β is a vector of coefficients to be estimated; and z is the vector of independent variables.
The partial likelihood function is modeled as an extension of the Cox proportional hazards model,[10] weighted by wij.
In this model, a patient who has suffered a competing risk is not removed from the risk set. This individual receives a
weight, where Ĝ is the nonparametric Kaplan-Meier[11] distribution of the censorship. The weight wij is decreasing due to the decay of the Kaplan-Meier curve. The distribution of the censorship is given by the pair (Ti,Ci) , and Ti is the time measured until the occurrence of the first competing event; and Ci=0 if it is observed the occurrence of some type of event and Ci=1 if no event occurs.[12] Thus, there is an inversion of the usual concepts of event and censorship.
In each instant tj,
in which the event of interest has been observed, the risk set is
composed of individuals who have not suffered any event until the time tj, receiving the weight wij=1; and those who have suffered a competing event before this time tj being weighted as wij≤1. Thus, given the occurrence of the event of interest in time tj, for each individual who has suffered a competing event in tj, the greater the distance between points ti and tj, the lower the weigh wij.[12] Results
The
causes of death of the 281 patients who died were analyzed. The most
common causes of death included infection mostly due to sepsis, acute
chest syndrome (ACS), overt stroke, sudden during crisis and organ
damage due to hepatic or renal failure. Thirteen patients died of
unrelated causes mostly due to trauma.
In this work, survival analysis was performed using the risk of sub-distribution regression model.[9]
These models consider the cumulative incidence function, using a
weighting factor for each individual considering all outcomes. Thus,
the individual who suffers from the competing event is not censored
receiving a weight that decreases gradually with time.
Eight models were implemented, one for each cause of death related to SCDA, as shown in Table 1:
Acute Chest Syndrome, Infection, Stroke, Cardiac Causes, Chronic Organ
Damage, Death During Crisis, Other (Splenic Sequestration, Hemolytic
Crisis or Hepatic Crisis) and Unknown.
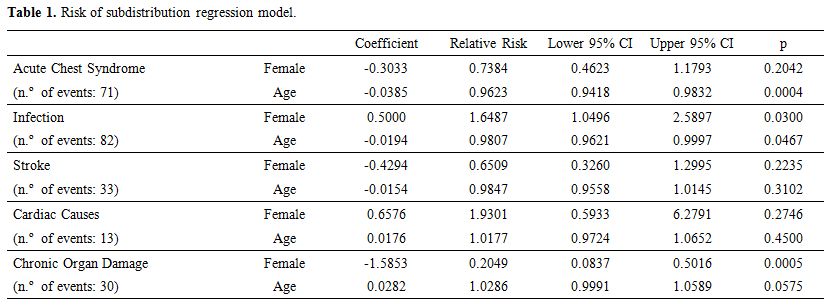 |
Table 1. Risk of subdistribution regression model.
|
It is observed that
the males are most vulnerable for death from chronic organ damage (p =
0.0005) while females are most vulnerable for death from infection
(p=0.03). Sex did not show a statistically significant association with
other causes of death. Age is significantly (p ≤ 0.05) associated with
death due to ACS, infection, and death during crisis. The increase of
one year in age corresponds to a 3.8% reduction in the risk of death by
ACS; 1.9% in the risk of death from infection; and 6.2% for death
during crisis. On the other hand, the increase of one year in age
implies an increase of 2.8% in the risk of death from chronic organ
damage, at a significance level of 10%.
Figure 1
shows the cumulative incidence function, where one can observe that the
lower survival is related to death from infection, followed by death
due to ACS. Compared to the model of competing risks, the independent
variables age and sex were significantly associated with the outcomes
with an asterisk.
Analyses were performed with the use of packages “cmprsk”[13] and “mstate”[14-16] of R software.[17]
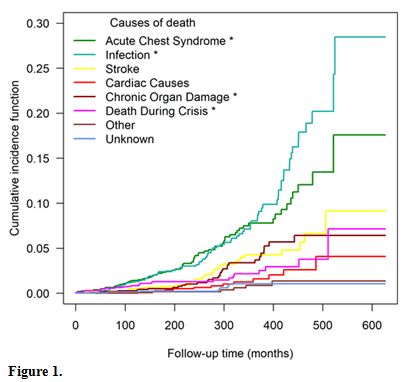 |
Figure 1 |
Discussion
Despite all Brazilian efforts, the mortality of patients with SCA is still very high in Brazil.[2,3]
These efforts included, among other things, newborn screening,
penicillin/antibiotic prophylaxis, vaccination including
anti-pneumococcus, anti-meningococcus, annual anti-influenza, blood
transfusion and transcranial doppler determinations.[3,5,18] In this study, we used the competitive risk analysis to evaluate the causes of mortality among our cohort.
A
competing risk is an event whose occurrence either precludes the
occurrence of another event under examination or fundamentally alters
the probability of occurrence of this other event, so it is an ideal
model to analyze causes of death in a specific disease with multiple
possible causes of death as in SCD.[6] It was used before in other chronic disease but never is SCD in Brazil before.[19]
The
competitive risk model was used because it is adequate in survival
analysis when there are mutually exclusive events, that is when the
occurrence of one event prevents another event occurring. In the
article, events are deaths from various causes. The cumulative
incidence function (represented by the graph) evaluates for each
patient the probability of occurrence of a specific event before a
certain time t. The risk sub-distribution model estimates the effect of
independent variables for each specific event, considering the presence
of competitive risks.
The reasons for the relatively low death
rate of females due to chronic organ damage are unknown. Possibilities
include gender differences in nitric oxide availability,[20] and the influence of the X-chromosome linked hemoglobin (Hb) F gene[21]
which may be protective against organ damage in females. However,
Dover’s et al. study was not confirmed by additional studies. In
addition, the increase in Hb F by the X-chromosome is minimal in
comparison to other studies indicating that Hb F has to be as high as ≥
8% to be effective. Other determinants of survival such as
hyperviscosity, alpha genotypes, and beta haplotypes could not be
determined at HEMORIO.
Conclusions
Mortality
among patients with SCD using the competing risks of death was
evaluated for the first time in a single institution in Rio de Janeiro,
Brazil.
In this article, we used the subdistribution hazard
function which evaluates the effect of covariates on the cumulative
incidence function for each of the competitive events. This modeling is
advantageous because it makes no assumption about the independence of
competitive events.
References
- Ware RE, de Montalembert M, Tshilolo L, Abboud MR. Sickle cell disease. Lancet. 2017;390(10091):311-23. https://doi.org/10.1016/S0140-6736(17)30193-9
- Piel FB, Steinberg MH, Rees DC. Sickle Cell Disease. N Engl J Med. 2017;377(3):305. https://doi.org/10.1056/NEJMra1510865
- Ballas SK. Sickle Cell Pain, 2nd Edition. Washington DC: International Association for the Study of Pain; 2014.
- Crowder M. Competing Risks. London: Chapman and Hall/CRC; 2001. https://doi.org/10.1201/9781420035902
- Lobo
CLC, Nascimento EMD, Jesus LJC, Freitas TG, Lugon JR, Ballas SK.
Mortality in children, adolescents and adults with sickle cell anemia
in Rio de Janeiro, Brazil. Rev Bras Hematol Hemoter. 2018;40(1):37-42. https://doi.org/10.1016/j.bjhh.2017.09.006
- Gooley
TA, Leisenring W, Crowley J, Storer BE. Estimation of failure
probabilities in the presence of competing risks: new representations
of old estimators. Stat Med. 1999;18(6):695-706. https://doi.org/10.1002/(SICI)1097-0258(19990330)18:6<695::AID-SIM60>3.0.CO;2-O
- Carvalho
M, S., Andreozzi V, L., Codeço C, T., Campos D, P., Barbosa M, T., S.,
Shimakura S, E. . Análise de sobrevivência: teoria e aplicações em
saúde. 2nd ed. Rio de Janeiro: Fiocruz; 2011.
- Kalbfleisch JD, Prentice RL. The Statistical Analysis of Failure Time Data. Hoboken: Wiley Interscience; 1980.
- Fine JP, Gray RJ. A proportional hazards model for the subdistribution of a competing risk. J Am Stat Assoc. 1999;94:496-509. https://doi.org/10.1080/01621459.1999.10474144
- Cox
DR. Regression Models and Life-Tables (with discussion). Journal of the
Royal Statistical Society Series B. 1972;34(2):187-220. https://doi.org/10.1111/j.2517-6161.1972.tb00899.x
- Kaplan EL, Meier P. Nonparametric estimation for incomplete observations. J Am Stat Assoc. 1958;53:457-81. https://doi.org/10.1080/01621459.1958.10501452
- Pintilie M. Competing Risks: A Practical Perspective. Sussex, England: John Wiley & Sons; 2006. https://doi.org/10.1002/9780470870709
- Gray B. cmprsk: Subdistribution Analysis of Competing Risks. R package version 2.2-7. 2014.
- Putter
H, Fiocco M, Geskus RB. Tutorial in biostatistics: competing risks and
multi-state models. Stat Med. 2007;26(11):2389-430. https://doi.org/10.1002/sim.2712 PMid:17031868
- de
Wreede LC, Fiocco M, Putter H. An R package for the analysis of
competing risks and multi-state models. J Stat Softw. 2011;38:1-30. https://doi.org/10.18637/jss.v038.i07
- de
Wreede LC, Fiocco M, Putter H. The mstate package for estimation and
prediction in non- and semi-parametric multi-state and competing risks
models. Comput Methods Programs Biomed. 2010;99(3):261-74. https://doi.org/10.1016/j.cmpb.2010.01.001 PMid:20227129
- R
Core Team. R: A language and environment for statistical computing. R
Foundation for Statistical Computing Vienna, Austria2017 [Available
from: http://www.R-project.org
- Lobo
CL, Ballas SK, Domingos AC, Moura PG, do Nascimento EM, Cardoso GP, et
al. Newborn screening program for hemoglobinopathies in Rio de Janeiro,
Brazil. Pediatr Blood Cancer. 2014;61(1):34-9. https://doi.org/10.1002/pbc.24711 PMid:24038856
- Baena-Diez
JM, Penafiel J, Subirana I, Ramos R, Elosua R, Marin-Ibanez A, et al.
Risk of Cause-Specific Death in Individuals With Diabetes: A Competing
Risks Analysis. Diabetes Care. 2016;39(11):1987-95. https://doi.org/10.2337/dc16-0614 PMid:27493134
- Gladwin
MT, Schechter AN, Ognibene FP, Coles WA, Reiter CD, Schenke WH, et al.
Divergent nitric oxide bioavailability in men and women with sickle
cell disease. Circulation. 2003;107(2):271-8. https://doi.org/10.1161/01.CIR.0000044943.12533.A8 PMid:12538427
- Dover
GJ, Smith KD, Chang YC, Purvis S, Mays A, Meyers DA, et al. Fetal
hemoglobin levels in sickle cell disease and normal individuals are
partially controlled by an X-linked gene located at Xp22.2. Blood.
1992;80(3):816-24. PMid:1379090
[TOP]