Enrica Antonia Martino1, Giuseppe Mele2, Ernesto Vigna1, Fortunato Morabito3 and Massimo Gentile1,4.
1 Hematology Unit, Azienda Ospedaliera Annunziata, Cosenza, Italy.
2 Haematology and Transplant Unit, Ospedale Antonio Perrino, Brindisi, Italy.
3 Gruppo Amici Dell'Ematologia Foundation-GrADE, Reggio Emilia, Italy.
4 Department of Pharmacy, Health and Nutritional Science, University of Calabria, Rende, Italy.
Correspondence to:
Massimo Gentile, MD, Hematology Unit, AO of Cosenza, Italy; 87100
Cosenza, Italy; viale della Repubblica snc, Tel.:
+39-0984-681329. Fax: +39-0984-681329. E-mail:
massim.gentile@tiscali.it.
Published: January 01, 2025
Received: October 31, 2024
Accepted: December 16, 2024
Mediterr J Hematol Infect Dis 2025, 17(1): e2025006 DOI
10.4084/MJHID.2025.006
This is an Open Access article distributed
under the terms of the Creative Commons Attribution License
(https://creativecommons.org/licenses/by-nc/4.0),
which permits unrestricted use, distribution, and reproduction in any
medium, provided the original work is properly cited.
|
Abstract
Multiple
myeloma (MM) is a heterogeneous disease, with MM patients experiencing
different clinical outcomes depending on the disease’s biological
features. Novel insights into the molecular mechanisms of MM have led
to the introduction of sophisticated drugs, which dramatically improved
patient treatment and survival. To date, young patients with newly
diagnosed MM could experience a median overall survival (OS) of 10
years. Nevertheless, a small proportion of patients still undergoes
early disease progression and death. Indeed, cases defined as
ultra-high-risk MM (uHRMM) and high-risk MM (HRMM) are destined for a
worse outcome, with an OS of 2-3 and 3-5 years, respectively. In this
regard, current risk stratification systems failed to identify this
subset of patients better. The application of existing risk models has
led to the identification of extremely heterogeneous categories of
patients, and they have not taken into account biological and clinical
differences. The concept of HRMM was initially formalised in 2015.
Since then, a great effort has been made to identify those parameters
whose presence pone MM patients at higher risk of developing an early
relapse. The simultaneous presence of 2 or more unfavourable
cytogenetic abnormalities, the identification of an extramedullary
disease or the detection of circulating plasma cells, as well as
high-risk gene expression profiling (GEP) signature, have shown to be
well related to a worse outcome and are going to be incorporated into
new prognostic systems. The introduction of the Individualised Risk
Model for Multiple Myeloma (IRMMa) marks a significant advancement in
the management of HRMM by integrating genomic and clinical data to
tailor treatment strategies. This model demonstrates improved
prognostic accuracy compared to traditional staging systems and
emphasises the importance of personalised treatment approaches. The
implementation of these advanced tools is essential for enhancing
precision medicine in MM and improving outcomes for patients in
high-risk categories.
|
Introduction
Multiple
myeloma (MM) has been regarded as a single disease entity,
characterised by an inferior overall survival (OS) in patients compared
to the general population. Nevertheless, considerable heterogeneity in
clinical presentation, treatment response, and outcome is evident in
daily clinical practice.[1] Event-free survival (EFS)
can vary dramatically, ranging from barely three years for
“ultra-high-risk” patients to up to ten years for “standard-risk”
patients, who fortunately represent the majority of cases.[2]
This underscores the critical importance of employing comprehensive
risk stratification to accurately identify patients at the highest risk
of disease progression, enabling them to benefit from tailored
therapeutic regimens.[3]
The concept of
high-risk multiple myeloma (HRMM) was initially formalised in 2015 with
the introduction of the Revised International Staging System (R-ISS),
which integrated parameters related to both tumour burden and disease
biology.[4] Over the years, advances in understanding
the pathogenic mechanisms of the disease, along with the development of
seminal diagnostic tools, have led to the validation and incorporation
of novel prognostic factors into clinical practice. These developments
have further evolved the definition of "high-risk" MM (HR-MM) patients.
Cytogenetic Abnormalities
In 2003, the introduction of the International Staging System (ISS)[5]
revolutionised the prognostication of MM, incorporating serum albumin
and beta-2-microglobulin (β2-M) levels as surrogates of tumour burden
and largely replacing the historical Durie-Salmon staging system.[6]
As evidence accumulated on the significant role of cytogenetic
abnormalities (CAs) in MM disease progression, particularly those
detected by fluorescence in situ
hybridisation (FISH), the ISS was empowered by the International
Myeloma Working Group (IMWG) to include high-risk cytogenetic lesions,
specifically translocation (4;14), translocation (14;16), and the
deletion 17p (del17p)].[4] This led to the development
of Revised-ISS (R-ISS), which also considered lactate dehydrogenase
(LDH) serum levels, reflecting increased proliferation and more
aggressive disease behaviour.[7]
The R-ISS
stratified patients into three risk categories (stages I, II, and III),
providing a more refined prognostic framework for both progression-free
survival (PFS) and OS. Nevertheless, the R-ISS exhibits some
limitations, including the exclusion of additional factors that
negatively impact the disease course. One such factor is chromosome 1
aberrations, particularly gain or amplification of 1q (gain1q/amp1q).
Gain1q refers to MM cells harbouring one extra copy of 1q, while amp1q
involves more than three copies.[8] The prevalence of
1q abnormalities increases with disease progression, from monoclonal
gammopathy of undetermined significance (MGUS) (0–20%) to
relapsed/refractory (RR) MM (≥50%),[8] suggesting a role in the dynamic process of clonal evolution and drug resistance.[9] Gene expression profiling (GEP) studies have identified key genes within the 1q21 band, such as CKS1B, ADAR, IL6R, ILF2, PSMD4, and MCL1, which are implicated in MM pathogenesis and therapy resistance, even in the era of novel agents.[10]
With
mounting evidence of the detrimental impact of 1q abnormalities on
prognosis, 1q abnormalities are now recognised as high-risk features in
newer staging systems. In 2019, the Intergroupe Francophone du Myelome
(IFM) included +1q and other clinically relevant CAs in their
definition of high-risk cytogenetics in MM.[11] The Myeloma Genome Project (MGP) also identified amp1q and bi-allelic TP53 inactivation as high-risk factors in patients classified as ISS III.[12]
In 2022, the Mayo Clinic proposed a new risk stratification model based
on five factors, including +1q, to better stratify patients into three
risk categories, building upon the R-ISS framework.[13]
This system has improved discriminatory power, particularly in the
R-ISS II group, which includes more than 60% of patients, further
stratifying them into intermediate-low and intermediate-high risk
categories.
Additionally, the European Myeloma Network (EMN)
introduced the R2-ISS, a 6-factor, 4-tier risk system, including ISS II
and III, del(17p), LDH levels, t(4;14), and +1q.[14]
The strength of this scoring system lies in its enhanced discriminative
capacity, especially within the R-ISS II group, which comprises over
60% of MM patients. This system further refines risk stratification by
subdividing this large R-ISS II group into intermediate-low and
intermediate-high categories. Such differentiation is critical because
it allows for more tailored prognostic assessments and therapeutic
strategies, addressing the heterogeneity within this sizeable subset of
patients and offering a more precise prediction of clinical outcomes.
The main risk stratification systems are shown in Table 1.
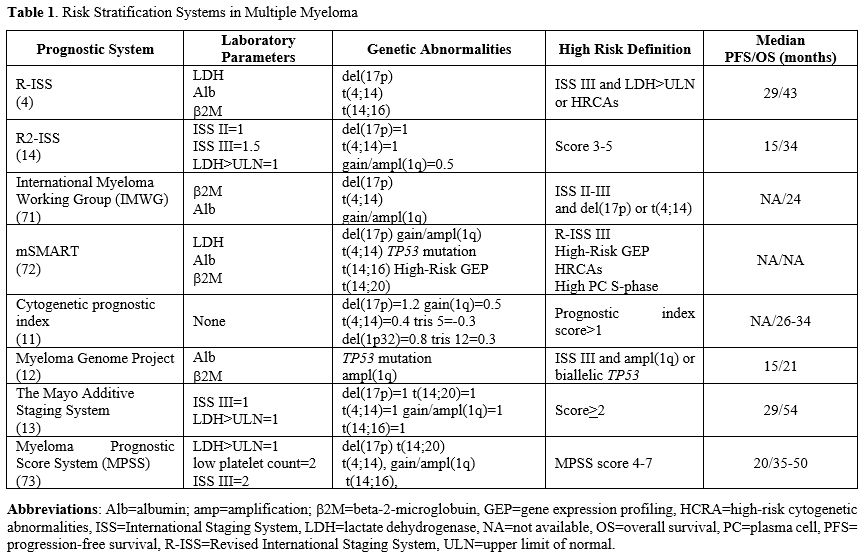 |
- Table 1. Risk Stratification Systems in Multiple Myeloma.
|
Despite
these advancements, current definitions of high-risk profiles remain
somewhat restrictive and oversimplified. Not all high-risk alterations
carry the same prognostic weight. For instance, Perrot et al.[15]
investigated the prognostic impact of del(17p), t(4;14), del(1 p32),
1q21 gain, and trisomies 3, 5, and 21 in a cohort of newly diagnosed MM
patients (NDMM). Six key cytogenetic abnormalities were identified, and
a prognostic index (PI) was developed, assigning weighted scores to
each abnormality. The study highlighted the poor prognosis conferred by
del(17p) abnormality, which is known to determine a poor prognosis
either alone or in combination with other adverse cytogenetic lesions.
Del(17p) is a recurrent cytogenetic abnormality detected in up to 80%
of relapsed/refractory MM (RRMM) cases, but rarely at the disease
onset, determining an aggressive disease course and poor outcomes.[16]
While the adverse prognostic role of del(17p) is well-established,
debates remain regarding the optimal threshold for its clinical
significance. The currently accepted 20% cut-off may not fully capture
its prognostic relevance, with some studies suggesting that a higher
threshold of 55-60% may be more appropriate.[15] A
more refined understanding of the prognostic role of del(17p) considers
not only the presence of the deletion but also the mutational status of
the TP53 gene, which is located on chromosome 17p(13.1) and encodes the tumour suppressor protein p53.[17] Mutations in TP53,
particularly when occurring in a biallelic manner, significantly worsen
prognosis, highlighting the need for comprehensive genetic assessment
to inform risk better stratification.[17] Biallelic inactivation of TP53, a condition referred to as “double-hit” TP53, is a potent marker of adverse prognosis compared to wild-type or monoallelic inactivation.[18]
Circulating Plasma Cells
Plasma
cell leukaemia (PCL), historically the most aggressive form of
monoclonal gammopathy, was originally defined by the presence of both
>20% circulating plasma cells (cPCs) and an absolute count >2×109/L of PCs.[19]
However, in 2021, the evidence demonstrated that the presence of ≥5%
circulating PCs in MM patients carried a similarly poor prognosis to
PCL. This finding led to a redefinition of PCL, reducing the threshold
for cPCs from 20% to 5%.[20] In 2023, the threshold
was further lowered to 2%, as studies showed that MM patients with
2-20% cPCs had significantly shorter PFS and OS than those patients
with <2%. Notably, patients with 2-5% cPCs exhibited outcomes
similar to those with 5-20% cPCs, reinforcing the idea that elevated
cPCs levels represent ultra-high-risk MM rather than a distinct
clinical entity.[21] Over time, advances in
laboratory techniques have underscored the prognostic value of cPCs.
The slide-based immunofluorescence assay, which required fluorescence
microscopy and was labour-intensive, has been largely replaced by
multiparameter flow cytometry (MFC). This technique provides a more
sensitive and reproducible method for quantifying cPCs.[22]
Further advancements have come with next-generation flow cytometry
(NGF), which could identify the presence of CPCs and enable the
detection of cPCs at much lower thresholds of 10-5-10-6.[23]
Several studies have already shown that the presence of cPCs has
highlighted the association between the presence of cPCs and poor
outcomes in both MM and related conditions, such as amyloidosis.[24,25]
Similarly, patients with MGUS and smouldering MM (sMM) who harbour ≥5%
cPCs are at an increased risk of progression to a symptomatic MM.[26] In a study by Garces et al.,[27]
using NGF to measure cPCs in 374 MM patients enrolled in the
GEM2012MENOS65 and GEM2014MAIN trials, higher percentages of cPCs were
associated with inferior PFS. In multivariable analyses, the cut-off of
0.01% cPCs retained its independent prognostic value alongside other
factors such as ISS, LDH, and cytogenetics. Similarly, a study by the
Greek group found that patients with cPCs above 2 x 10-4 had a higher risk of progressing, irrespective of the ISS stage, cytogenetic abnormalities, or the induction therapy utilised.[28]
Bertamini et al. further analysed the cPCs of 410 MM patients enrolled
in the FORTE clinical trials, identifying a threshold of 0.07% as
optimal for distinguishing patients at higher risk of poor outcomes.[29]
Several efforts have been made to incorporate cPCs quantification into
existing staging systems, such as the R-ISS. It has been demonstrated
that the presence of ≥5 cPCs/μL, as detected by MFC, can identify
patients classified as R-ISS I and II who are at risk of poor outcomes,
comparable to those in stage III.[30] Similarly,
Galieni et al. established that baseline cPCs detection serves as a
useful tool for better stratifying R-ISS II patients.[31]
These
findings firmly support the prognostic significance of cPCs as a
remarkable prognostic marker for identifying high-risk MM patients.
Extramedullary Disease
Two
distinct forms of extramedullary disease (EMD) are currently recognised
in MM: 1) EMD involving soft tissues, such as the liver, lymph nodes,
spleen, kidneys, breast, pleura, meninges, testes or skin, and 2)
paraskeletal (PS) disease characterised by tumour masses arising from
skeletal lesions. Only the EMD is recognised as a high-risk MM.
Observational studies have reported an increased incidence of both
forms of EMD during disease relapse[32] and following allogeneic transplantation with dose-reduced intensity conditioning regimens.[33,34]
Regardless of when EMD occurs during the disease course, its presence
is consistently associated with significantly worse outcomes.[35]
Historically, in the era of chemotherapy, EMD was linked to
significantly worse prognoses. However, the introduction of high-dose
melphalan followed by autologous stem cell transplantation (ASCT) has
shown survival benefits for patients with EMD, partially overcoming its
adverse prognostic implications.[36] A study
conducted by the EBMT Chronic Malignancies Working Party highlighted
the differential prognosis between patients with EMD and those with PS
disease.[37] Notably, patients with EMD had
significantly shorter 3-year-PFS and OS rates after ASCT compared to
those with PS disease or without plasmacytomas.
Furthermore,
tandem transplantation did not confer any additional benefit in this
patient population. Moreover, tandem transplantation did not confer any
additional benefit in this setting of patients. The role of allogeneic
stem cell transplantation (allo-SCT) in EMD has also been investigated.
A study including 155 patients demonstrated that the presence of EMD
prior to allo-SCT was significantly associated with an unfavourable
prognosis, with a median OS of fewer than 8 months. However, allo-SCT
was capable of inducing long-term remissions, even in patients
harbouring high-risk cytogenetic lesions and multiorgan involvement.[38]
In the era of novel therapies, further insights into the prognostic
impact of EMD have emerged. A recent Italian meta-analysis, which
included eight trials, investigated NDMM patients’ clinical features,
outcomes, and responses to new drug regimens. The analysis revealed no
significant difference in median PFS between patients with and without
EMD in a multivariable model. However, OS was worse in patients with
EMD. This meta-analysis suggests that novel therapies may help mitigate
the negative prognostic impact of extramedullary disease.[39]
Accurate
assessment of EMD, as well as response to treatment, requires advanced
imaging techniques, such as magnetic resonance imaging (MRI) and
18-fluorodeoxyglucose positron emission tomography/computed tomography
(FDG-PET/CT). MRI is particularly effective in detecting lesions and in
assessing the extent of soft-tissue disease in both EMD and PS
soft-tissue involvement, especially in cases of suspected spinal or
central nervous system involvement. This radiological technique may
accurately locate the level of the lesion and quantify its extent and
the degree of damage.[40] PET/TC, known for its
higher sensitivity, offers additional information on the metabolic
activity of lesions and serves as a valuable prognostic tool. Several
retrospective studies have demonstrated the detrimental impact of a
positive PET/TC on survival. In 2011, Zamagni et al. conducted a
prospective study evaluating the prognostic relevance of PET/CT in 192
NDMM patients who underwent thalidomide-dexamethasone induction
followed by double ASCT. The analysis revealed that a standardised
uptake value (SUV) greater than 4.2 and persistent pathologic uptake
after ASCT were independent predictors of reduced PFS.[41]
A subsequent prospective study by the French group in 2017 compared MRI
and PET/TC in NDMM patients treated with
lenalidomide-bortezomib-dexamethasone (RVD), with or without ASCT,
followed by lenalidomide maintenance. This prospective analysis failed
to demonstrate any difference in detecting bone lesions at diagnosis
between the two image techniques but showed that PET/CT normalisation
before maintenance was associated with improved PFS and OS.[42] The CASSIOPET trial, a companion study of the CASSIOPEIA trial,[43]
investigated PFS differences between baseline PET-negative and
PET-positive patients in both arms of the trial, confirming that
baseline PET negativity is associated with better survival outcomes.[44]
Minimal Residual Disease
Clinical
trials and meta-analyses have established that the achievement of
minimal residual disease (MRD) is strongly linked to improved survival
outcomes in MM. The concept of MRD was first introduced by IMWG in
2015, referring to those patients who, despite achieving a complete
response, still harbour a low level of residual disease.[45] MRD
assessment is typically performed using MFC and molecular biology
techniques with a sensitivity between 10-5 and 10-6.[46]
A meta-analysis including fourteen studies investigating the impact of
MRD on PFS and 12 studies on OS was conducted to evaluate the clinical
significance of MRD detection in NDMM patients.[47]
The results confirmed the predictive value of MRD negativity, as it was
associated with significantly better PFS. In a separate analysis, MRD
negativity was also evaluated in patients with RRMM and NDMM patients
who were transplant-ineligible and treated with daratumumab-based
regimens. MRD-negative status. In this analysis, patients achieving a
complete response or better (≥CR) who also reached MRD-negative status
demonstrated superior outcomes in terms of PFS.[48]
Sustained MRD negativity for at least 6 months, even in patients
harbouring high-risk cytogenetic lesions, has been associated with
significant improvements in both PFS and OS, reinforcing the idea that
undetectable MRD can serve as a key treatment endpoint for this
high-risk population.[49,50] Conversely, the loss of MRD negativity has been correlated with an increased risk of progression or death.[51]
In light of these findings, several trials are now incorporating MRD
negativity as a primary endpoint and are investigating treatment
strategies tailored to MRD status. The MASTER trial, a multicentre
phase II study, demonstrated that achieving MRD negativity after
induction, ASCT, and consolidation allowed patients to avoid
maintenance with lenalidomide.[52] Similarly, a British study[53]
is investigating the potential for de-escalating therapy in patients
who achieve MRD negativity post-ASCT. The phase III IFM MIDAS trial is
evaluating the role of single versus
double ASCT in the context of MRD-driven treatment strategies, while
the DRAMMATIC trial (ClinicalTrials.gov Identifier: NCT04052880) is
exploring the possibility of discontinuing maintenance therapy after 2
years if MRD negativity is achieved. These studies collectively support
the growing role of MRD as a crucial endpoint in MM treatment,
especially as novel therapies and personalised approaches continue to
evolve.
Functional High-Risk Myeloma
Functional
high-risk MM (FHRMM) refers to patients who exhibit adverse disease
biology that becomes evident after the failure of first-line therapy.
This includes those who relapse within 18 months of treatment
initiation and/or within 12 months of frontline ASCT,[54]
encompassing both primary refractory patients and those with early
relapse. In addition to R-ISS, other parameters, including a suboptimal
response to first-line therapy or inappropriate induction therapy,
should considered in identifying FHRMM patients, though these factors
may underestimate the true risk. Emerging research highlights the role
of the tumour micro-environment in both MM pathogenesis and treatment
response. A recent study on bone marrow samples from NDMM patients
revealed that the T-cell repertoire undergoes significant changes over
time with treatment. In particular, a decrease in early memory T cells
and an increase in senescent T-cell numbers were observed.[55]
Alrasheed et al. examined bone marrow samples from NDMM patients before
treatment induction and again 100 days post-ASCT, focusing on T-Regs.
They found that a higher frequency of T-Regs was associated with poorer
PFS and OS.[56]
Additionally, increased levels of naïve and terminally differentiated T cells post-ASCT were linked to worsening prognosis.[57]
Disruptions in the immune microenvironment, including altered
expression of inhibitory receptors on cytotoxic T cells and
downregulation of costimulatory receptors, such as CD226, can
contribute to disease progression.[58] Studies also
show that NK cells play a pivotal role in preserving response to
therapy, particularly in patients who undergo ASCT. Higher NK cell
count is associated with better outcomes and a higher rate of MRD
negativity, while impairments in NK cell number and function increase
the risk of relapse.[59,60]
Gene expression
profiling (GEP) assays represent valuable tools in predicting FHRMM.
Several genes involved in MM cell metabolism and transduction
signalling pathways, such as IL-6/JAK/STAT3,[61,62]
were found to be overexpressed in FHRMM cases. Moreover, disruptions in
DNA damage repair pathways and mutations in the TP53 gene occur more
frequently in FHRMM patients compared to those who relapse later in the
disease course.[63] A recent study involving 104 NDMM patients used the GEP70/UAMS70 assay to predict relapse-free survival (RFS) and OS.[64]
The main endpoints included relapse-free survival (RFS) and OS. An RNA
microarray platform was used to identify low-risk and high-risk
cohorts. The study demonstrated that patients with higher GEP scores
experienced higher relapse rates within one year and poorer OS,
regardless of their baseline FISH.
High-Risk Prediction in the Era of Artificial Intelligence
Recent
advances in transcriptomic, exomic, and whole-genome sequencing have
identified new genomic alterations and molecular signatures in MM. The
CoMMpass study revealed distinct molecular subgroups, with
approximately 25% of patients transitioning to high-risk categories at
first relapse.[65] Similarly, Walker et al.
emphasised the prognostic impact of single-nucleotide mutations (SNVs)
and APOBEC mutational signatures alongside the International Staging
System (ISS).[66] Whole-genome sequencing further
highlighted the role of APOBEC signatures and structural variants, such
as chromothripsis, in driving MM progression and survival outcomes.[67,68]
Building
on these findings, recent approaches in MM classification and
individualised risk prediction now leverage artificial intelligence
(AI)-based models that integrate clinical, genomic, and therapeutic
data to generate more precise and adaptable risk classifiers.[69,70]
These AI-driven models offer significant improvements over traditional
systems, such as the ISS and its revisions (R-ISS, R2-ISS), by
incorporating a broader array of variables, including genomic markers
such as TP53 mutations, 1q21
gain, chromothripsis, and NSD2 translocations. This allows for a more
refined stratification of patients, offering a personalised approach to
prognosis and treatment planning.[69,70] In the study
by Maura et al., the Individualised Risk Model for Multiple Myeloma
(IRMMa) was developed, integrating clinical, genomic, and therapeutic
data from 1,933 newly diagnosed multiple myeloma (NDMM) patients to
account for the heterogeneity of outcomes, where overall survival (OS)
ranges from months to over a decade.[70] This model incorporates 20 key genomic features, such as 1q21 gain/amp, TP53
loss, and APOBEC mutational signatures, and demonstrated superior
accuracy compared to existing models like the ISS, R-ISS, and R2-ISS,
with a concordance index (c-index) for OS of 0.726. Furthermore, the
study identified 12 distinct genomic clusters, validated using data
from the GMMG-HD6 clinical trial, allowing the IRMMa model to predict
treatment efficacy, particularly regarding high-dose melphalan followed
by ASCT.[70]
One key finding from this study was
the IRMMa model's ability to incorporate time-dependent factors,
including treatment strategies such as ASCT and maintenance therapies,
to predict patient outcomes better. Patients with high-risk genomic
profiles showed a poorer response to these therapies, highlighting the
need for personalised treatment approaches based on genomic insights.
Additionally, patients classified as high-risk by the IRMMa model -
especially those harbouring TP53 mutations,
1q21 gains, or chromothripsis - were found to have a significantly
increased risk of death. These high-risk patients often experienced
relapse within 18 months of initial treatment, and their OS was
substantially lower compared to those with lower-risk profiles. In
these cases, the IRMMa model predicted that the likelihood of death
within three years was markedly higher in patients classified as having
high-risk genomic signatures. For example, patients with TP53 loss or chromothripsis had a more than 50% higher risk of death compared to those without these mutations.
The
IRMMa model is not only more accurate than traditional staging systems
but also adaptable, providing clinicians with tools to tailor therapies
based on each patient’s genomic profile, significantly reducing the
risk of death for those in higher-risk categories.
Conclusions
Multiple
myeloma is an extremely complex disease, with clinical outcomes that
are strictly dependent on its underlying biological characteristics.
Advances in our understanding of the molecular mechanisms driving MM
have significantly improved patient treatment and survival.
Nevertheless, further effort is needed to standardise the definition of
high-risk patients and identify therapeutic strategies aimed at
improving outcomes. Current risk stratification systems, such as R-ISS,
do not fully capture all high-risk MM cases. Therefore, integration
with novel prognostic factors is expected to enhance risk
identification (Table 2).
Chromosome 1 abnormalities, for example, have been shown to exert an
unfavourable prognostic impact, and FISH cytogenetics at baseline
should routinely include these disruptions.
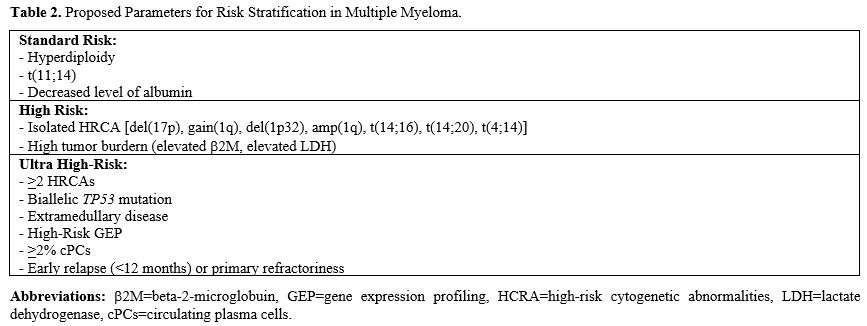 |
- Table 2. Proposed Parameters for Risk Stratification in Multiple Myeloma.
|
The
detection of cPCs rate using MFC has emerged as a useful parameter in
identifying patients with aggressive disease, as demonstrated by many
retrospective analyses.[21,29]
However, validation and standardisation of this technique, especially
within prospective clinical trials, are recommended. Additionally,
FDG-PET/CT should be incorporated into routine baseline prognostic
evaluations, given its ability to provide topographic, quantitative,
and metabolic information about EMD, which is known to contribute to an
unfavourable prognosis.[41]
In this regard,
most data regarding FDG-PET/CT are derived from retrospective studies
with small populations, highlighting the need for prospective
evaluations.
There has also been growing interest in MRD
assessment, particularly due to the deeper responses obtained with
novel agents. MRD assessment, detected via NGS or NGF, has proven to be
a strong prognostic marker, serving as a good surrogate for both PFS
and OS. Open issues remain concerning the standardisation of NGF, the
optimal threshold for MRD negativity, the appropriate time points for
analysis, and the definition of sustained MRD negativity and loss of
MRD-negative status. Ongoing prospective clinical trials will
undoubtedly address these questions.
The development of the IRMMa[69,70]
is a promising step forward in the management of HRMM. By integrating
genomic data with clinical and treatment variables, the model offers a
more personalised approach to prognosis and treatment planning,
significantly improving the limitations of traditional systems like the
R-ISS.
It could lead to improved outcomes for patients,
particularly those who may not benefit from standard treatment
protocols. However, implementing such a model in clinical practice will
require widespread access to genomic testing and the integration of
these data into clinical workflows. While the IRMMa model shows
significant potential, further validation in diverse patient
populations and real-world settings is crucial to confirm its utility
and accuracy. The integration of these advanced tools will be key to
advancing precision medicine in MM and improving outcomes for those in
the highest-risk categories.
References
- Kumar SK, Rajkumar SV. The multiple myelomas -
current concepts in cytogenetic classification and therapy. Nat Rev
Clin Oncol. 2018 Jul;15(7):409-421. doi: 10.1038/s41571-018-0018-y. https://doi.org/10.1038/s41571-018-0018-y PMid:29686421
- Ravi
P, Kumar SK, Cerhan JR, Maurer MJ, Dingli D, Ansell SM, Rajkumar SV.
Defining cure in multiple myeloma: a comparative study of outcomes of
young individuals with myeloma and curable hematologic malignancies.
Blood Cancer J. 2018 Feb 28;8(3):26. doi: 10.1038/s41408-018-0065-8. https://doi.org/10.1038/s41408-018-0065-8 PMid:29531285 PMCid:PMC5849889
- Goldschmidt
H, Lokhorst HM, Mai EK, van der Holt B, Blau IW, Zweegman S, Weisel KC,
Vellenga E, Pfreundschuh M, Kersten MJ, Scheid C, Croockewit S,
Raymakers R, Hose D, Potamianou A, Jauch A, Hillengass J, Stevens-Kroef
M, Raab MS, Broijl A, Lindemann HW, Bos GMJ, Brossart P, van Marwijk
Kooy M, Ypma P, Duehrsen U, Schaafsma RM, Bertsch U, Hielscher T,
Jarari L, Salwender HJ, Sonneveld P. Bortezomib before and after
high-dose therapy in myeloma: long-term results from the phase III
HOVON-65/GMMG-HD4 trial. Leukemia. 2018 Feb;32(2):383-390. doi:
10.1038/leu.2017.211. Epub 2017 Jul 4. https://doi.org/10.1038/leu.2017.211 PMid:28761118
- Palumbo
A, Avet-Loiseau H, Oliva S, Lokhorst HM, Goldschmidt H, Rosinol L, et
al.. Revised international staging system for multiple myeloma: A
report from international myeloma working group. J Clin Oncol (2015)
33(26):2863-9. doi: 10.1200/JCO.2015.61.2267 https://doi.org/10.1200/JCO.2015.61.2267 PMid:26240224 PMCid:PMC4846284
- Greipp PR, San Miguel JF, Durie BG, et al. International staging system for multiple myeloma. J Clin Oncol. 2005;23:3412-3420. https://doi.org/10.1200/JCO.2005.04.242 PMid:15809451
- Durie
BG, Salmon SE. A clinical staging system for multiple myeloma.
Correlation of measured myeloma cell mass with presenting clinical
features, response to treatment, and survival. Cancer.
1975;36(3):842-854. https://doi.org/10.1002/1097-0142(197509)36:3<842::AID-CNCR2820360303>3.0.CO;2-U PMid:1182674
- Caro
J, Al Hadidi S, Usmani S, Yee AJ, Raje N, Davies FE. How to treat
high-risk myeloma at diagnosis and relapse. Am Soc Clin Oncol Educ Book
(2021) 41):291-309. doi: 10.1200/EDBK_320105. https://doi.org/10.1200/EDBK_320105 PMid:34010042
- Bisht
K, Walker B, Kumar SK, et al. Chromosomal 1q21 abnormalities in
multiple myeloma: a review of translational, clinical research, and
therapeutic strategies. Expert Rev Hematol. 2021;14:1099-1114. https://doi.org/10.1080/17474086.2021.1983427 PMid:34551651
- Neben
K, Jauch A, Hielscher T, et al. Progression in smoldering myeloma is
independently determined by the chromosomal abnormalities del(17p),
t(4;14), gain 1q, hyperdiploidy, and tumor load. J Clin Oncol Off J Am
Soc Clin Oncol. 2013;31:4325-4332. https://doi.org/10.1200/JCO.2012.48.4923 PMid:24145347
- Burroughs
Garcìa J, Eufemiese RA, Storti P, et al. Role of 1q21 in Multiple
Myeloma: From Pathogenesis to Possible Therapeutic Targets. Cells.
2021;10:1360. https://doi.org/10.3390/cells10061360 PMid:34205916 PMCid:PMC8227721
- Perrot
A, Lauwers-Cances V, Tournay E, Hulin C, Chretien ML, Royer B, et al.
Development and validation of a cytogenetic prognostic index predicting
survival in multiple myeloma. J Clin Oncol (2019) 37(19):1657-65. doi:
10.1200/JCO.18.00776. https://doi.org/10.1200/JCO.18.00776 PMid:31091136 PMCid:PMC6804890
- Walker
BA, Mavrommatis K, Wardell CP, et al. A high-risk, Double-Hit, group of
newly diagnosed myeloma identified by genomic analysis. Leukemia.
2019;33:159-170. https://doi.org/10.1038/s41375-018-0196-8 PMid:29967379 PMCid:PMC6326953
- Abdallah
NH, Binder M, Rajkumar SV, Greipp PT, Kapoor P, Dispenzieri A, Gertz
MA, Baughn LB, Lacy MQ, Hayman SR, Buadi FK, Dingli D, Go RS, Hwa YL,
Fonder AL, Hobbs MA, Lin Y, Leung N, Kourelis T, Warsame R, Siddiqui
MA, Kyle RA, Bergsagel PL, Fonseca R, Ketterling RP, Kumar SK. A simple
additive staging system for newly diagnosed multiple myeloma. Blood
Cancer J. 2022 Jan 31;12(1):21. doi: 10.1038/s41408-022-00611-x. https://doi.org/10.1038/s41408-022-00611-x PMid:35102148 PMCid:PMC8803917
- D'Agostino
M, Cairns DA, Lahuerta JJ, et al. Second Revision of the International
Staging System (R2-ISS) for Overall Survival in Multiple Myeloma: A
European Myeloma Network (EMN) Report Within the HARMONY Project. J
Clin Oncol. 2022;JCO.21.02614.
- Perrot
A, Lauwers-Cances V, Tournay E, Hulin C, Chretien ML, Royer B, Dib M,
Decaux O, Jaccard A, Belhadj K, Brechignac S, Fontan J, Voillat L,
Demarquette H, Collet P, Rodon P, Sohn C, Lifermann F, Orsini-Piocelle
F, Richez V, Mohty M, Macro M, Minvielle S, Moreau P, Leleu X, Facon T,
Attal M, Avet-Loiseau H, Corre J. Development and Validation of a
Cytogenetic Prognostic Index Predicting Survival in Multiple Myeloma. J
Clin Oncol. 2019 Jul 1;37(19):1657-1665. doi: 10.1200/JCO.18.00776.
Epub 2019 May 15. https://doi.org/10.1200/JCO.18.00776 PMid:31091136 PMCid:PMC6804890
- Manier
S, Salem KZ, Park J, Landau DA, Getz G, Ghobrial IM. Genomic complexity
of multiple myeloma and its clinical implications. Nat Rev Clin Oncol.
2017 Feb;14(2):100-113. doi: 10.1038/nrclinonc.2016.122. Epub 2016 Aug
17.. https://doi.org/10.1038/nrclinonc.2016.122 PMid:27531699
- Herrero
A, Rojas E, Misiewicz-Krzeminska I, Krzeminski P, Gutiérrez N.
Molecular mechanisms of p53 deregulation in cancer: An overview in
multiple myeloma. IJMS (2016) 17(12):2003. doi: 10.3390/ijms17122003. https://doi.org/10.3390/ijms17122003 PMid:27916892 PMCid:PMC5187803
- Weinhold
N, Ashby C, Rasche L, Chavan SS, Stein C, Stephens OW, Tytarenko R,
Bauer MA, Meissner T, Deshpande S, Patel PH, Buzder T, Molnar G,
Peterson EA, van Rhee F, Zangari M, Thanendrarajan S, Schinke C, Tian
E, Epstein J, Barlogie B, Davies FE, Heuck CJ, Walker BA, Morgan GJ.
Clonal selection and double-hit events involving tumor suppressor genes
underlie relapse in myeloma. Blood. 2016 Sep 29;128(13):1735-44. doi:
10.1182/blood-2016-06-723007. Epub 2016 Aug 11. https://doi.org/10.1182/blood-2016-06-723007 PMid:27516441 PMCid:PMC5043128
- Kyle RA, Maldonado JE, Bayrd ED. Plasma cell leukemia. Report on 17 cases. Arch Intern Med. 1974;133(15):813-818. https://doi.org/10.1001/archinte.133.5.813 PMid:4821776
- Fernández
de Larrea C, Kyle R, Rosiñol L, Paiva B, Engelhardt M, Usmani S, Caers
J, Gonsalves W, Schjesvold F, Merlini G, Lentzch S, Ocio E, Garderet L,
Moreau P, Sonneveld P, Badros A, Gahrton G, Goldschmidt H, Tuchman S,
Einsele H, Durie B, Wirk B, Musto P, Hayden P, Kaiser M, Miguel JS,
Bladé J, Rajkumar SV, Mateos MV. Primary plasma cell leukemia:
consensus definition by the International Myeloma Working Group
according to peripheral blood plasma cell percentage. Blood Cancer J.
2021 Dec 2;11(12):192. doi: 10.1038/s41408-021-00587-0. PMID: 34857730;
PMCID: PMC8640034. https://doi.org/10.1038/s41408-21-00587-0 PMid:34857730 PMCid:PMC8640034
- Jelinek
T, Bezdekova R, Zihala D, Sevcikova T, Anilkumar Sithara A, Pospisilova
L, Sevcikova S, Polackova P, Stork M, Knechtova Z, Venglar O, Kapustova
V, Popkova T, Muronova L, Chyra Z, Hrdinka M, Simicek M, Garcés JJ,
Puig N, Cedena MT, Jurczyszyn A, Castillo JJ, Penka M, Radocha J,
Mateos MV, San-Miguel JF, Paiva B, Pour L, Rihova L, Hajek R. More Than
2% of Circulating Tumor Plasma Cells Defines Plasma Cell Leukemia-Like
Multiple Myeloma. J Clin Oncol. 2023 Mar 1;41(7):1383-1392. doi:
10.1200/JCO.22.01226. Epub 2022 Oct 31. PMID: 36315921; PMCID:
PMC9995102. https://doi.org/10.1200/JCO.22.01226 PMid:36315921 PMCid:PMC9995102
- Gonsalves
WI, Rajkumar SV, Gupta V, Morice WG, Timm MM, Singh PP, Dispenzieri A,
Buadi FK, Lacy MQ, Kapoor P, Gertz MA, Kumar SK. Quantification of
clonal circulating plasma cells in newly diagnosed multiple myeloma:
implications for redefining high-risk myeloma. Leukemia. 2014
Oct;28(10):2060-5. doi: 10.1038/leu.2014.98. Epub 2014 Mar 12. PMID:
24618735; PMCID: PMC4162866. https://doi.org/10.1038/leu.2014.98 PMid:24618735 PMCid:PMC4162866
- Sanoja-Flores
L, Flores-Montero J, Garcés JJ, Paiva B, Puig N, García-Mateo A, et
al.. Next generation flow for minimally-invasive blood characterization
of MGUS and multiple myeloma at diagnosis based on circulating tumor
plasma cells (CTPC). Blood Cancer J (2018) 8(12):117. doi:
10.1038/s41408-018-0153-9. https://doi.org/10.1038/s41408-018-0153-9 PMid:30455467 PMCid:PMC6242818
- Witzig
TE, Dhodapkar MV, Kyle RA, Greipp PR. Quantitation of circulating
peripheral blood plasma cells and their relationship to disease
activity in patients with multiple myeloma. Cancer. 1993 Jul
1;72(1):108-13. doi:
10.1002/1097-0142(19930701)72:1<108::aid-cncr2820720121>3.0.co;2-t. https://doi.org/10.1002/1097-0142(19930701)72:1<108::AID-CNCR2820720121>3.0.CO;2-T PMid:8508395
- Nowakowski
GS, Witzig TE, Dingli D, Tracz MJ, Gertz MA, Lacy MQ, Lust JA,
Dispenzieri A, Greipp PR, Kyle RA, Rajkumar SV. Circulating plasma
cells detected by flow cytometry as a predictor of survival in 302
patients with newly diagnosed multiple myeloma. Blood. 2005 Oct
1;106(7):2276-9. doi: 10.1182/blood-2005-05-1858. Epub 2005 Jun
16. https://doi.org/10.1182/blood-2005-05-1858 PMid:15961515 PMCid:PMC1895270
- Bianchi
G, Kyle RA, Larson DR, Witzig TE, Kumar S, Dispenzieri A, Morice WG,
Rajkumar SV. High levels of peripheral blood circulating plasma cells
as a specific risk factor for progression of smoldering multiple
myeloma. Leukemia. 2013 Mar;27(3):680-5. doi: 10.1038/leu.2012.237.
Epub 2012 Aug 20. https://doi.org/10.1038/leu.2012.237 PMid:22902364 PMCid:PMC3597230
- Garcés
JJ, Cedena MT, Puig N, Burgos L, Perez JJ, Cordon L, Flores-Montero J,
Sanoja-Flores L, Calasanz MJ, Ortiol A, Blanchard MJ, Rios R, Martin J,
Martínez-Martinez R, Bargay J, Sureda A, de la Rubia J, Hernandez MT,
Rodriguez-Otero P, de la Cruz J, Orfao A, Mateos MV, Martinez-Lopez J,
Lahuerta JJ, Rosiñol L, Blade J, San-Miguel JF, Paiva B. Circulating
Tumor Cells for the Staging of Patients With Newly Diagnosed
Transplant-Eligible Multiple Myeloma. J Clin Oncol. 2022 Sep
20;40(27):3151-3161. doi: 10.1200/JCO.21.01365. Epub 2022 Jun 6. https://doi.org/10.1200/JCO.21.01365 PMid:35666958
- Kostopoulos
IV, Ntanasis-Stathopoulos I, Rousakis P, Eleutherakis-Papaiakovou E,
Panteli C, Malandrakis P, Angelis N, Kanellias N, Orologas-Stavrou N,
Papanota A, Fotiou D, Migkou M, Gavriatopoulou M, Kastritis E,
Tsitsilonis O, Terpos E, Dimopoulos MA. Circulating Plasma Cells in
Newly Diagnosed Multiple Myeloma: Prognostic and More. J Clin Oncol.
2023 Jan 20;41(3):708-710. doi: 10.1200/JCO.22.01606. Epub 2022 Sep
30. https://doi.org/10.1200/JCO.22.01606 PMid:36179274
- Bertamini
L, Oliva S, Rota-Scalabrini D, Paris L, Morè S, Corradini P, Ledda A,
Gentile M, De Sabbata G, Pietrantuono G, Pascarella A, Tosi P, Curci P,
Gilestro M, Capra A, Galieni P, Pisani F, Annibali O, Monaco F,
Liberati AM, Palmieri S, Luppi M, Zambello R, Fazio F, Belotti A,
Tacchetti P, Musto P, Boccadoro M, Gay F. High Levels of Circulating
Tumor Plasma Cells as a Key Hallmark of Aggressive Disease in
Transplant-Eligible Patients With Newly Diagnosed Multiple Myeloma. J
Clin Oncol. 2022 Sep 20;40(27):3120-3131. doi: 10.1200/JCO.21.01393.
Epub 2022 Jun 6. https://doi.org/10.1200/JCO.21.01393 PMid:35666982
- Gonsalves
WI, Jevremovic D, Nandakumar B, Dispenzieri A, Buadi FK, Dingli D, Lacy
MQ, Hayman SR, Kapoor P, Leung N, Fonder A, Hobbs M, Hwa YL, Muchtar E,
Warsame R, Kourelis TV, Russell S, Lust JA, Lin Y, Go RS, Siddiqui MA,
Kyle RA, Gertz MA, Rajkumar SV, Kumar SK. Enhancing the R-ISS
classification of newly diagnosed multiple myeloma by quantifying
circulating clonal plasma cells. Am J Hematol. 2020 Mar;95(3):310-315.
doi: 10.1002/ajh.25709. Epub 2020 Jan 8. https://doi.org/10.1002/ajh.25709 PMid:31867775 PMCid:PMC7724649
- Galieni
P, Travaglini F, Vagnoni D, Ruggieri M, Caraffa P, Bigazzi C, Falcioni
S, Picardi P, Mazzotta S, Troiani E, Dalsass A, Mestichelli F, Angelini
M, Camaioni E, Maravalle D, Angelini S, Pezzoni V. The detection of
circulating plasma cells may improve the Revised International Staging
System (R-ISS) risk stratification of patients with newly diagnosed
multiple myeloma. Br J Haematol. 2021 May;193(3):542-550. doi:
10.1111/bjh.17118. Epub 2021 Apr 1. https://doi.org/10.1111/bjh.17118 PMid:33792026
- Bladé
J, Fernández de Larrea C, Rosiñol L, Cibeira MT, Jiménez R, Powles R.
Soft-tissue plasmacytomas in multiple myeloma: incidence, mechanisms of
extramedullary spread, and treatment approach. J Clin Oncol. 2011 Oct
1;29(28):3805-12. doi: 10.1200/JCO.2011.34.9290. Epub 2011 Sep 6. https://doi.org/10.1200/JCO.2011.34.9290 PMid:21900099
- Pérez-Simón
JA, Sureda A, Fernández-Aviles F, Sampol A, Cabrera JR, Caballero D,
Martino R, Petit J, Tomás JF, Moraleda JM, Alegre A, Cañizo C, Brunet
S, Rosiñol L, Lahuerta J, Díez-Martín JL, León A, García A, Vazquez L,
Sierra J, San Miguel JF; Grupo Español de Mieloma. Reduced-intensity
conditioning allogeneic transplantation is associated with a high
incidence of extramedullary relapses in multiple myeloma patients.
Leukemia. 2006 Mar;20(3):542-5. doi: 10.1038/sj.leu.2404085. https://doi.org/10.1038/sj.leu.2404085 PMid:16408097
- Minnema
MC, van de Donk NW, Zweegman S, Hegenbart U, Schonland S, Raymakers R,
Zijlmans JM, Kersten MJ, Bos GM, Lokhorst HM. Extramedullary relapses
after allogeneic non-myeloablative stem cell transplantation in
multiple myeloma patients do not negatively affect treatment outcome.
Bone Marrow Transplant. 2008 May;41(9):779-84. doi:
10.1038/sj.bmt.1705982. Epub 2008 Jan 14. https://doi.org/10.1038/sj.bmt.1705982 PMid:18195681
- Varettoni
M, Corso A, Pica G, Mangiacavalli S, Pascutto C, Lazzarino M.
Incidence, presenting features and outcome of extramedullary disease in
multiple myeloma: a longitudinal study on 1003 consecutive patients.
Ann Oncol. 2010 Feb;21(2):325-330. doi: 10.1093/annonc/mdp329. Epub
2009 Jul 24. https://doi.org/10.1093/annonc/mdp329 PMid:19633044
- Wu
P, Davies FE, Boyd K, Thomas K, Dines S, Saso RM, Potter MN, Ethell ME,
Shaw BE, Morgan GJ. The impact of extramedullary disease at
presentation on the outcome of myeloma. Leuk Lymphoma. 2009
Feb;50(2):230-5. doi: 10.1080/10428190802657751. https://doi.org/10.1080/10428190802657751 PMid:19197724
- Gagelmann
N, Eikema DJ, Iacobelli S, Koster L, Nahi H, Stoppa AM, Masszi T,
Caillot D, Lenhoff S, Udvardy M, Crawley C, Arcese W, Mariette C,
Hunter A, Leleu X, Schipperus M, Delforge M, Pioltelli P, Snowden JA,
Itälä-Remes M, Musso M, van Biezen A, Garderet L, Kröger N. Impact of
extramedullary disease in patients with newly diagnosed multiple
myeloma undergoing autologous stem cell transplantation: a study from
the Chronic Malignancies Working Party of the EBMT. Haematologica. 2018
May;103(5):890-897. doi: 10.3324/haematol.2017.178434. Epub 2018 Feb 1.
PMID: 29419433; PMCID: PMC5927971.
- Rasche
L, Röllig C, Stuhler G, Danhof S, Mielke S, Grigoleit GU, Dissen L,
Schemmel L, Middeke JM, Rücker V, Schreder M, Schetelig J, Bornhäuser
M, Einsele H, Thiede C, Knop S. Allogeneic Hematopoietic Cell
Transplantation in Multiple Myeloma: Focus on Longitudinal Assessment
of Donor Chimerism, Extramedullary Disease, and High-Risk Cytogenetic
Features. Biol Blood Marrow Transplant. 2016 Nov;22(11):1988-1996. doi:
10.1016/j.bbmt.2016.08.024. Epub 2016 Aug 31. https://doi.org/10.1016/j.bbmt.2016.08.024 PMid:27590108
- Montefusco
V, Gay F, Spada S, De Paoli L, Di Raimondo F, Ribolla R, Musolino C,
Patriarca F, Musto P, Galieni P, Ballanti S, Nozzoli C, Cascavilla N,
Ben-Yehuda D, Nagler A, Hajek R, Offidani M, Liberati AM, Sonneveld P,
Cavo M, Corradini P, Boccadoro M. Outcome of paraosseous
extra-medullary disease in newly diagnosed multiple myeloma patients
treated with new drugs. Haematologica. 2020 Jan;105(1):193-200. doi:
10.3324/haematol.2019.219139. Epub 2019 Jun 20. https://doi.org/10.3324/haematol.2019.219139 PMid:31221778 PMCid:PMC6939525
- Dimopoulos
MA, Hillengass J, Usmani S, Zamagni E, Lentzsch S, Davies FE, Raje N,
Sezer O, Zweegman S, Shah J, Badros A, Shimizu K, Moreau P, Chim CS,
Lahuerta JJ, Hou J, Jurczyszyn A, Goldschmidt H, Sonneveld P, Palumbo
A, Ludwig H, Cavo M, Barlogie B, Anderson K, Roodman GD, Rajkumar SV,
Durie BG, Terpos E. Role of magnetic resonance imaging in the
management of patients with multiple myeloma: a consensus statement. J
Clin Oncol. 2015 Feb 20;33(6):657-64. doi: 10.1200/JCO.2014.57.9961.
Epub 2015 Jan 20. https://doi.org/10.1200/JCO.2014.57.9961 PMid:25605835
- Zamagni
E, Patriarca F, Nanni C, Zannetti B, Englaro E, Pezzi A, Tacchetti P,
Buttignol S, Perrone G, Brioli A, Pantani L, Terragna C, Carobolante F,
Baccarani M, Fanin R, Fanti S, Cavo M. Prognostic relevance of 18-F FDG
PET/CT in newly diagnosed multiple myeloma patients treated with
up-front autologous transplantation. Blood. 2011 Dec 1;118(23):5989-95.
doi: 10.1182/blood-2011-06-361386. Epub 2011 Sep 6. Erratum in: Blood.
2012 Sep 13;120(11):2349. https://doi.org/10.1182/blood-2011-06-361386 PMid:21900189
- Moreau
P, Attal M, Caillot D, Macro M, Karlin L, Garderet L, Facon T,
Benboubker L, Escoffre-Barbe M, Stoppa AM, Laribi K, Hulin C, Perrot A,
Marit G, Eveillard JR, Caillon F, Bodet-Milin C, Pegourie B, Dorvaux V,
Chaleteix C, Anderson K, Richardson P, Munshi NC, Avet-Loiseau H,
Gaultier A, Nguyen JM, Dupas B, Frampas E, Kraeber-Bodere F.
Prospective Evaluation of Magnetic Resonance Imaging and
[18F]Fluorodeoxyglucose Positron Emission Tomography-Computed
Tomography at Diagnosis and Before Maintenance Therapy in Symptomatic
Patients With Multiple Myeloma Included in the IFM/DFCI 2009 Trial:
Results of the IMAJEM Study. J Clin Oncol. 2017 Sep 1;35(25):2911-2918.
doi: 10.1200/JCO.2017.72.2975. Epub 2017 Jul 7. https://doi.org/10.1200/JCO.2017.72.2975 PMid:28686535 PMCid:PMC5578392
- Moreau
P, Attal M, Hulin C, Arnulf B, Belhadj K, Benboubker L, Béné MC, Broijl
A, Caillon H, Caillot D, Corre J, Delforge M, Dejoie T, Doyen C, Facon
T, Sonntag C, Fontan J, Garderet L, Jie KS, Karlin L, Kuhnowski F,
Lambert J, Leleu X, Lenain P, Macro M, Mathiot C, Orsini-Piocelle F,
Perrot A, Stoppa AM, van de Donk NW, Wuilleme S, Zweegman S, Kolb B,
Touzeau C, Roussel M, Tiab M, Marolleau JP, Meuleman N, Vekemans MC,
Westerman M, Klein SK, Levin MD, Fermand JP, Escoffre-Barbe M,
Eveillard JR, Garidi R, Ahmadi T, Zhuang S, Chiu C, Pei L, de Boer C,
Smith E, Deraedt W, Kampfenkel T, Schecter J, Vermeulen J, Avet-Loiseau
H, Sonneveld P. Bortezomib, thalidomide, and dexamethasone with or
without daratumumab before and after autologous stem-cell
transplantation for newly diagnosed multiple myeloma (CASSIOPEIA): a
randomised, open-label, phase 3 study. Lancet. 2019 Jul
6;394(10192):29-38. doi: 10.1016/S0140-6736(19)31240-1. Epub 2019 Jun
3. Erratum in: Lancet. 2024 Aug 31;404(10455):e3. doi:
10.1016/S0140-6736(19)31403-5. https://doi.org/10.1016/S0140-6736(19)31403-5 PMid:31208815
- Kraeber-Bodéré
F, Zweegman S, Perrot A, Hulin C, Caillot D, Facon T, Leleu X, Belhadj
K, Itti E, Karlin L, Bailly C, Levin MD, Minnema MC, Jamet B,
Bodet-Milin C, De Keizer B, Béné MC, Avet-Loiseau H, Sonneveld P, Pei
L, Rigat F, De Boer C, Vermeulen J, Kampfenkel T, Lambert J, Moreau P.
Prognostic value of positron emission tomography/computed tomography in
transplant-eligible newly diagnosed multiple myeloma patients from
CASSIOPEIA: the CASSIOPET study. Haematologica. 2023 Feb
1;108(2):621-626. doi: 10.3324/haematol.2021.280051. https://doi.org/10.3324/haematol.2021.280051 PMid:36263839 PMCid:PMC9890028
- Kumar
S, Paiva B, Anderson KC, Durie B, Landgren O, Moreau P, et al.
International Myeloma Working Group consensus criteria for response and
minimal residual disease assessment in multiple myeloma. Lancet Oncol.
2016;17:e328-46. https://doi.org/10.1016/S1470-2045(16)30206-6 PMid:27511158
- Stetler-Stevenson
M, Paiva B, Stoolman L, Lin P, Jorgensen JL, Orfao A, Van Dongen J,
Rawstron AC. Consensus guidelines for myeloma minimal residual disease
sample staining and data acquisition. Cytometry B Clin Cytom. 2016
Jan;90(1):26-30. doi: 10.1002/cyto.b.21249. Epub 2015 Jul 6. https://doi.org/10.1002/cyto.b.21249 PMid:25907102 PMCid:PMC7511978
- Munshi
NC, Avet-Loiseau H, Rawstron AC, Owen RG, Child JA, Thakurta A,
Sherrington P, Samur MK, Georgieva A, Anderson KC, Gregory WM.
Association of Minimal Residual Disease With Superior Survival Outcomes
in Patients With Multiple Myeloma: A Meta-analysis. JAMA Oncol. 2017
Jan 1;3(1):28-35. doi: 10.1001/jamaoncol.2016.3160. https://doi.org/10.1001/jamaoncol.2016.3160 PMid:27632282 PMCid:PMC5943640
- Cavo
M, San-Miguel J, Usmani SZ, Weisel K, Dimopoulos MA, Avet-Loiseau H,
Paiva B, Bahlis NJ, Plesner T, Hungria V, Moreau P, Mateos MV, Perrot
A, Iida S, Facon T, Kumar S, van de Donk NWCJ, Sonneveld P, Spencer A,
Krevvata M, Heuck C, Wang J, Ukropec J, Kobos R, Sun S, Qi M, Munshi N.
Prognostic value of minimal residual disease negativity in myeloma:
combined analysis of POLLUX, CASTOR, ALCYONE, and MAIA. Blood. 2022 Feb
10;139(6):835-844. doi: 10.1182/blood.2021011101. https://doi.org/10.1182/blood.2021011101 PMid:34289038 PMCid:PMC8832474
- Goicoechea
I, Puig N, Cedena MT, Burgos L, Cordón L, Vidriales MB, Flores-Montero
J, Gutierrez NC, Calasanz MJ, Ramos MM, Lara-Astiaso D, Vilas-Zornoza
A, Alignani D, Rodriguez I, Sarvide S, Alameda D, Garcés JJ, Rodriguez
S, Fresquet V, Celay J, Garcia-Sanz R, Martinez-Lopez J, Oriol A, Rios
R, Martin-Sanchez J, Martinez-Martinez R, Sarra J, Hernandez MT, de la
Rubia J, Krsnik I, Moraleda JM, Palomera L, Bargay J, Martinez-Climent
JA, Orfao A, Rosiñol L, Mateos MV, Lahuerta JJ, Blade J, San Miguel J,
Paiva B. Deep MRD profiling defines outcome and unveils different modes
of treatment resistance in standard- and high-risk myeloma. Blood. 2021
Jan 7;137(1):49-60. doi: 10.1182/blood.2020006731. https://doi.org/10.1182/blood.2020006731 PMid:32693406
- San-Miguel
J, Avet-Loiseau H, Paiva B, Kumar S, Dimopoulos MA, Facon T, Mateos MV,
Touzeau C, Jakubowiak A, Usmani SZ, Cook G, Cavo M, Quach H, Ukropec J,
Ramaswami P, Pei H, Qi M, Sun S, Wang J, Krevvata M, DeAngelis N, Heuck
C, Van Rampelbergh R, Kudva A, Kobos R, Qi M, Bahlis NJ. Sustained
minimal residual disease negativity in newly diagnosed multiple myeloma
and the impact of daratumumab in MAIA and ALCYONE. Blood. 2022 Jan
27;139(4):492-501. doi: 10.1182/blood.2020010439. https://doi.org/10.1182/blood.2020010439 PMid:34269818 PMCid:PMC8796656
- Diamond
B, Korde N, Lesokhin AM, Smith EL, Shah U, Mailankody S, Hultcrantz M,
Hassoun H, Lu SX, Tan C, Rustad EH, Maura F, Maclachlan K, Peterson T,
Derkach A, Devlin S, Landau HJ, Scordo M, Chung DJ, Shah GL, Lahoud O,
Thoren K, Murata K, Ramanathan L, Arcila ME, Ho C, Roshal M, Dogan A,
Giralt SA, Landgren O. Dynamics of minimal residual disease in patients
with multiple myeloma on continuous lenalidomide maintenance: a
single-arm, single-centre, phase 2 trial. Lancet Haematol. 2021
Jun;8(6):e422-e432. doi: 10.1016/S2352-3026(21)00130-7. https://doi.org/10.1016/S2352-3026(21)00130-7 PMid:34048681
- Costa
LJ, Chhabra S, Medvedova E, Dholaria BR, Schmidt TM, Godby KN,
Silbermann R, Dhakal B, Bal S, Giri S, D'Souza A, Hall A, Hardwick P,
Omel J, Cornell RF, Hari P, Callander NS. Daratumumab, Carfilzomib,
Lenalidomide, and Dexamethasone With Minimal Residual Disease
Response-Adapted Therapy in Newly Diagnosed Multiple Myeloma. J Clin
Oncol. 2022 Sep 1;40(25):2901-2912. doi: 10.1200/JCO.21.01935. Epub
2021 Dec 13. https://doi.org/10.1200/JCO.21.01935 PMid:34898239
- Yong
K., Royle K.L., Ramasamy K., Parrish C., Hockaday A., Asher S., Drayson
M.T., de Tute R.M., Jenner M.W., Kaiser M.F., et al. Risk-adapted
therapy directed according to response (RADAR, UK-MRA Myeloma
XV)-Comparing MRD-guided treatment escalation and de-escalation
strategies in patients with newly diagnosed myeloma suitable for stem
cell transplantation. Blood. 2022;140((Suppl. S1)):1844-1846. doi:
10.1182/blood-2022-168842. https://doi.org/10.1182/blood-2022-168842
- Banerjee
R, Cicero KI, Lee SS, Cowan AJ. Definers and drivers of functional
high-risk multiple myeloma: insights from genomic, transcriptomic, and
immune profiling. Front Oncol. 2023 Oct 2;13:1240966. doi:
10.3389/fonc.2023.1240966. PMID: 37849816; PMCID: PMC10577204. https://doi.org/10.3389/fonc.2023.1240966 PMid:37849816 PMCid:PMC10577204
- Visram
A, Dasari S, Anderson E, Kumar S, Kourelis TV. Relapsed multiple
myeloma demonstrates distinct patterns of immune microenvironment and
Malignant cell-mediated immunosuppression. Blood Cancer J (2021)
11(3):45. doi: 10.1038/s41408-021-00440-4. https://doi.org/10.1038/s41408-021-00440-4 PMid:33649314 PMCid:PMC7921408
- Alrasheed
N, Lee L, Ghorani E, Henry JY, Conde L, Chin M, et al..
Marrow-infiltrating regulatory T cells correlate with the presence of
dysfunctional CD4+PD-1+ Cells and inferior survival in patients with
newly diagnosed multiple myeloma. Clin Cancer Res (2020)
26(13):3443-54. doi: 10.1158/1078-0432.Ccr-19-1714. https://doi.org/10.1158/1078-0432.CCR-19-1714 PMid:32220887
- Parmar
H, Gertz M, Anderson EI, Kumar S, Kourelis TV. Microenvironment immune
reconstitution patterns correlate with outcomes after autologous
transplant in multiple myeloma. Blood Adv (2021) 5(7):1797-804. doi:
10.1182/bloodadvances.2020003857. https://doi.org/10.1182/bloodadvances.2020003857 PMid:33787859 PMCid:PMC8045512
- Minnie
SA, Kuns RD, Gartlan KH, Zhang P, Wilkinson AN, Samson L, et al..
Myeloma escape after stem cell transplantation is a consequence of
T-cell exhaustion and is prevented by TIGIT blockade. Blood (2018)
132(16):1675-88. doi: 10.1182/blood-2018-01-825240. https://doi.org/10.1182/blood-2018-01-825240 PMid:30154111
- Keruakous
AR, Asch A, Aljumaily R, Zhao D, Yuen C. Prognostic impact of natural
killer cell recovery on minimal residual disease after autologous stem
cell transplantation in multiple myeloma. Transpl Immunol (2022)
71:101544. doi: 10.1016/j.trim.2022.101544. https://doi.org/10.1016/j.trim.2022.101544 PMid:35093506 PMCid:PMC10434759
- Merino
AM, Mehta RS, Luo X, Kim H, De For T, Janakiram M, et al.. Early
adaptive natural killer cell expansion is associated with decreased
relapse after autologous transplantation for multiple myeloma.
Transplant Cell Ther (2021) 27(4):310.e1-.e6. doi:
10.1016/j.jtct.2020.10.023. https://doi.org/10.1016/j.jtct.2020.10.023 PMid:33836870 PMCid:PMC9776115
- Soekojo
CY, Chung TH, Furqan MS, Chng WJ. Genomic characterization of
functional high-risk multiple myeloma patients. Blood Cancer J (2022)
12(1):24. doi: 10.1038/s41408-021-00576-3. https://doi.org/10.1038/s41408-021-00576-3 PMid:35102139 PMCid:PMC8803925
- Poos
AM, Giesen N, Catalano C, Paramasivam N, Huebschmann D, John L, et al..
Comprehensive comparison of early relapse and end-stage relapsed
refractory multiple myeloma. Blood (2020) 136(Supplement 1):1-. doi:
10.1182/blood-2020-141611. https://doi.org/10.1182/blood-2020-141611
- D'Agostino
M, Zaccaria GM, Ziccheddu B, Rustad EH, Genuardi E, Capra A, et al..
Early relapse risk in patients with newly diagnosed multiple myeloma
characterized by next-generation sequencing. Clin Cancer Res (2020)
26(18):4832-41. doi: 10.1158/1078-0432.CCR-20-0951. https://doi.org/10.1158/1078-0432.CCR-20-0951 PMid:32616499
- Manasanch
E, Berrios D, Fountain E, Claussen CM, Chaung T, Kaufman GP, et al..
Gene expression profiling predicts relapse-free and overall survival in
newly diagnosed myeloma patients treated with novel therapies. Br J
Haematol (2021) 192(4):e115-e20. doi: 10.1111/bjh.17344 https://doi.org/10.1111/bjh.17344 PMid:33486754
- Skerget
S, Penaherrera D, Chari A, et al: Genomic basis of multiple myeloma
subtypes from the MMRF CoMMpass study. medRxiv, 2021. https://doi.org/10.1101/2021.08.02.21261211
- Walker
BA, Boyle EM, Wardell CP, et al: Mutational spectrum, copy number
changes, and outcome: Results of a sequencing study of patients with
newly diagnosed myeloma. J Clin Oncol 33:3911-3920, 2015.
- Maura
F, Petljak M, Lionetti M, et al: Biological and prognostic impact of
APOBEC-induced mutations in the spectrum of plasma cell dyscrasias and
multiple myeloma cell lines. Leukemia 32:1044-1048, 2018. https://doi.org/10.1038/leu.2017.345 PMid:29209044 PMCid:PMC5886048
- Rustad
EH, Yellapantula VD, Glodzik D, et al: Revealing the impact of
structural variants in multiple myeloma. Blood Cancer Discov 1:258-273,
2020 https://doi.org/10.1158/2643-3230.BCD-20-0132 PMid:33392515 PMCid:PMC7774871
- Neri
P, Lee H, Bahlis NJ. Artificial Intelligence Individualized Risk
Classifier in Multiple Myeloma. J Clin Oncol. 2024 Apr
10;42(11):1207-1210. https://doi.org/10.1200/JCO.23.02781 PMid:38452316
- Maura
F, Rajanna AR, Ziccheddu B, Poos AM, Derkach A, Maclachlan K, Durante
M, Diamond B, Papadimitriou M, Davies F, Boyle EM, Walker B, Hultcrantz
M, Silva A, Hampton O, Teer JK, Siegel EM, Bolli N, Jackson GH, Kaiser
M, Pawlyn C, Cook G, Kazandjian D, Stein C, Chesi M, Bergsagel L, Mai
EK, Goldschmidt H, Weisel KC, Fenk R, Raab MS, Van Rhee F, Usmani S,
Shain KH, Weinhold N, Morgan G, Landgren O. Genomic Classification and
Individualized Prognosis in Multiple Myeloma. J Clin Oncol. 2024 Apr
10;42(11):1229-1240. https://doi.org/10.1200/JCO.23.01277 PMid:38194610 PMCid:PMC11095887
- Chng
WJ, Dispenzieri A, Chim CS, Fonseca R, Goldschmidt H, Lentzsch S,
Munshi N, Palumbo A, Miguel JS, Sonneveld P, Cavo M, Usmani S, Durie
BG, Avet-Loiseau H; International Myeloma Working Group. IMWG consensus
on risk stratification in multiple myeloma. Leukemia. 2014
Feb;28(2):269-77. doi: 10.1038/leu.2013.247. Epub 2013 Aug 26. https://doi.org/10.1038/leu.2013.247 PMid:23974982
- Mikhael
JR, Dingli D, Roy V, Reeder CB, Buadi FK, Hayman SR, Dispenzieri A,
Fonseca R, Sher T, Kyle RA, Lin Y, Russell SJ, Kumar S, Bergsagel PL,
Zeldenrust SR, Leung N, Drake MT, Kapoor P, Ansell SM, Witzig TE, Lust
JA, Dalton RJ, Gertz MA, Stewart AK, Rajkumar SV, Chanan-Khan A, Lacy
MQ; Mayo Clinic. Management of newly diagnosed symptomatic multiple
myeloma: updated Mayo Stratification of Myeloma and Risk-Adapted
Therapy (mSMART) consensus guidelines 2013. Mayo Clin Proc. 2013
Apr;88(4):360-76. doi: 10.1016/j.mayocp.2013.01.019. Erratum in: Mayo
Clin Proc. 2013 Jul;88(7):777. Stewart, Keith [corrected to Stewart, A
Keith]. https://doi.org/10.1016/j.mayocp.2013.01.019 PMid:23541011
- Mao
X, Yan W, Mery D, Liu J, Fan H, Xu J, Xu Y, Sui W, Deng S, Zou D, Du C,
Yi S, van Rhee F, Barlogie B, Shaughnessy JD Jr, Anderson KC, Zhan F,
Qiu L, An G. Development and validation of an individualized and
weighted Myeloma Prognostic Score System (MPSS) in patients with newly
diagnosed multiple myeloma. Am J Hematol. 2024 Apr;99(4):523 https://doi.org/10.1002/ajh.27207 PMid:38247315